Presentation
Scalable Reduced-Order Modeling for Three-Dimensional Turbulent Flow
SessionResearch Posters Display
DescriptionA neural network-based reduced order modeling method for three-dimensional turbulent flow simulation is proposed. This method was implemented as the scalable distributed learning on Fugaku. Our method constitutes a dimensional reduction using a convolutional-autoencoder-like neural network and the time evolution prediction using long short-term memory networks. The time evolution of the turbulent three-dimensional flow (e.g., Re=2.8×10^6) could be simulated at a significantly lower cost (approximately four orders of magnitude) without a major loss in accuracy. Using the single core memory group, our implementation shows 370 GFLOPS (24.28% of the peak performance) for the entire training loop and 753 GFLOPS (24.28% of the peak performance) for the convolution kernel. Our implementation scales up to 25,250 computational nodes (1,212,000 cores). Thus it shows 72.9 % of weak scaling performance (7.8 PFLOPS) for the entire training loop. On the other hand, the convolution routine shows 100.8% of weak scaling performance (113 PFLOPS).
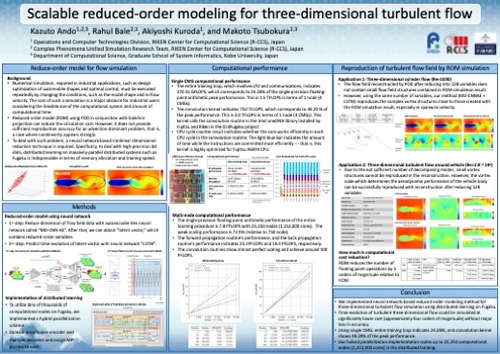
Event Type
Posters
Research Posters
TimeTuesday, 14 November 202310am - 5pm MST
LocationDEF Concourse
TP
XO/EX
Archive
view