Presentation
A Reinforcement Learning-Based Backfilling Strategy for HPC Batch Jobs
DescriptionHigh Performance Computing (HPC) systems are essential for various scientific fields, and effective job scheduling is crucial for their performance. Traditional backfilling techniques, such as EASY-backfilling, rely on user-submitted runtime estimates, which can be inaccurate and lead to suboptimal scheduling. This poster presents RL-Backfiller, a novel reinforcement learning (RL) based approach to improve HPC job scheduling. Our method incorporates RL to make better backfilling decisions, independent of user-submitted runtime estimates. We trained RL-Backfiller on the synthetic Lublin-256 workload and tested it on the real SDSC-SP2 1998 workload. We show how RLBackfilling can learn effective backfilling strategies and outperform traditional EASY-backfilling and other heuristic combinations via trial-and-error on existing job traces. Our evaluation results show up to 17x better scheduling performance (based on average bounded job slowdown) compared to EASY-backfilling
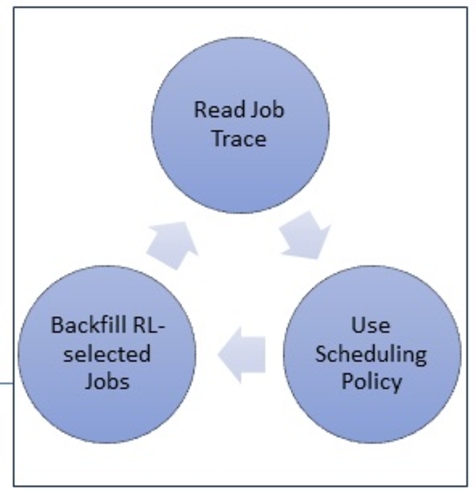
Event Type
ACM Student Research Competition: Graduate Poster
ACM Student Research Competition: Undergraduate Poster
Posters
TimeTuesday, 14 November 202310am - 5pm MST
LocationDEF Concourse
TP
XO/EX
Archive
view